The security vulnerabilities of off-chip memory can undermine the confidentiality and integrity necessary for deep neural network (DNN) accelerators that process private data and make critical decisions. This work illustrates such vulnerabilities and proposes an effective, hardware-friendly defense solution that provides memory security for DNN accelerators.
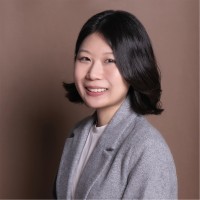
Speaker
Kyungmi Lee
Kyungmi Lee received a Ph.D. degree in Electrical Engineering and Computer Science from MIT in May 2024. She is currently a Postdoctoral Associate at Prof. Anantha Chandrakasan’s group. Her research interests are security and energy-efficiency of machine learning hardware systems.
Explore
MIT Engineers Advance Toward a Fault-tolerant Quantum Computer
Adam Zewe | MIT News
Researchers achieved a type of coupling between artificial atoms and photons that could enable readout and processing of quantum information in a few nanoseconds.
New Method Efficiently Safeguards Sensitive AI Training Data
Adam Zewe | MIT News
The approach maintains an AI model’s accuracy while ensuring attackers can’t extract secret information.
The Road to Gate-All-Around CMOS
Monday, April 14, 2025 | 10:00 AM to 11:00 AM
In-Person
Haus Room (36-428)
50 Vassar Street Cambridge, MA